By: Sarah Johnson
Predictive modeling is revolutionizing business strategies and operations by leveraging AI and data analytics to anticipate trends and optimize efficiency, offering a critical advantage in today’s fiercely competitive market where cost efficiency is vital to success.
At the heart of every successful enterprise lies the constant drive to minimize expenses while maximizing efficiency. This pursuit has never been more crucial than in today’s competitive markets, where every penny saved can mean the difference between thriving and merely surviving. Business leaders are increasingly turning to innovative approaches to tackle the challenge of reducing operational costs, and predictive modeling stands at the forefront of this movement.
By leveraging historical data, advanced algorithms can predict future trends with remarkable accuracy, enabling businesses to make informed decisions and streamline their processes. Bruce Shi, who studied finance and data analytics, delves into the transformative potential of predictive modeling in cutting operational costs, exploring its applications, benefits, and the profound impact it can have on the bottom line of any organization.
Understanding Predictive Modeling
Predictive modeling, a cornerstone of Artificial Intelligence (AI), involves using historical data to make informed predictions about future outcomes. It employs various types of models, such as regression, classification, and time series analysis.
“Regression models predict continuous outcomes, like sales figures, based on input variables,” says Bruce Shi. “Classification models categorize data into classes, such as predicting whether a customer will buy a product or not. Time series models forecast future trends based on historical data patterns.”
The efficacy of predictive modeling hinges on the quality and quantity of data available. Accurate predictions rely on clean, relevant, and sufficient data points. Without these, models may produce unreliable results. Thus, organizations keen on reducing operational costs recognize the need to invest in robust data collection and processing mechanisms.
Businesses across industries now leverage predictive modeling to gain a competitive edge. It forecasts customer behaviors and market trends and helps optimize operations. In the quest to streamline expenses, companies are turning to predictive modeling as a powerful tool to anticipate future costs and strategically allocate resources.
The Benefits of Predictive Modeling in Cost Reduction
Predictive modeling offers a proactive approach to cost reduction by providing insights into future outcomes. Businesses can forecast demand, optimize inventory levels, and minimize surplus or shortage scenarios by analyzing historical data patterns. This capability is especially beneficial for industries reliant on efficient inventory management, such as retail and manufacturing.
Another area where predictive modeling shines is in maintenance scheduling. By predicting equipment failures before they occur, companies can implement preventive maintenance, reducing downtime and the associated costs. For instance, airlines use predictive modeling in the transportation sector to anticipate engine maintenance needs, ensuring flights run smoothly and avoiding costly delays.
“Predictive modeling aids in risk management, identifying potential hazards or inefficiencies in operations,” Notes Bruce Shi.
This proactive approach allows businesses to mitigate risks before they escalate, thereby avoiding costly consequences. For insurance companies, predictive modeling helps assess risks accurately, leading to more precise pricing and reduced financial exposure. The benefits of predictive modeling in cost reduction are vast and varied.
From optimizing inventory to minimizing maintenance costs and enhancing risk management, businesses can realize substantial savings by leveraging predictive analytics. This section highlights the multifaceted advantages of incorporating predictive modeling into cost-reduction strategies, paving the way for a more efficient and profitable operation.
Implementing Predictive Modeling Strategies
Implementing predictive modeling for cost reduction involves several key steps to ensure its effectiveness. The first crucial step is data collection. Organizations need to gather relevant data from various sources, such as sales records, customer demographics, and operational metrics. This data must then undergo preprocessing, where inconsistencies are resolved, missing values are addressed, and outliers are identified. Clean and well-prepared data is essential for accurate predictive models.
Next, selecting the appropriate algorithms is pivotal. Different algorithms suit different types of predictive tasks. For instance, linear regression may be suitable for predicting sales trends, while decision trees could be effective for customer segmentation. The choice of algorithm should align with the specific cost-reduction goals of the business.
Once the algorithms are chosen, model evaluation methods come into play. This step ensures the model’s accuracy and reliability. Techniques such as cross-validation and metrics like Mean Absolute Error (MAE) or Root Mean Square Error (RMSE) help assess how well the model performs on unseen data.
Successful implementation also involves continuous monitoring and refinement of the predictive models. As businesses evolve, so do their data and operational needs. Regularly updating and improving the models ensures they remain relevant and effective in reducing operational costs.
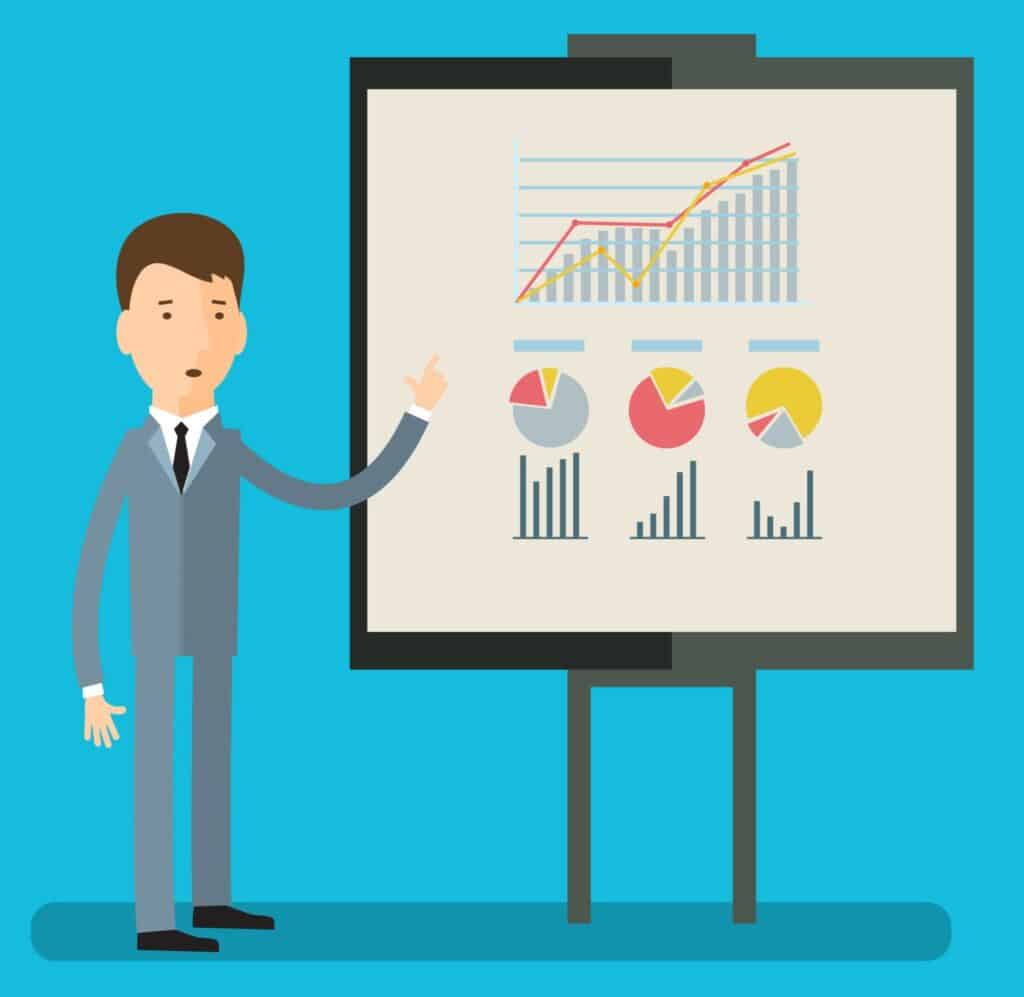
Photo: DepositPhotos.com
Overcoming Challenges and Limitations
Despite its benefits, predictive modeling for cost reduction comes with its own set of challenges and limitations. One common challenge is the availability and quality of data. Insufficient or poor-quality data can hinder the accuracy of predictive models, leading to unreliable cost-reduction forecasts.
“Businesses can employ strategies such as data augmentation, where additional relevant data is sourced or generated to supplement existing datasets. This helps improve the robustness and accuracy of the models,” says Shi.
Another challenge is the complexity of predictive models. Overly complex models may be challenging to interpret and implement, especially for businesses with limited technical expertise. Simplifying models without compromising their predictive power is a strategy to overcome this hurdle. By focusing on the most crucial variables and features, businesses can create more straightforward yet effective models for cost reduction.
Ethical considerations also play a significant role in predictive modeling. Biases in data or algorithms can lead to unfair outcomes, impacting certain groups disproportionately. It is essential for businesses to be aware of these biases and take steps to mitigate them. Transparent model development, regular audits, and diverse teams working on the models can help address ethical concerns and promote fairness.
Navigating these challenges requires a balanced approach, where businesses prioritize data quality, model simplicity, and ethical considerations. By doing so, organizations can unlock the full potential of predictive modeling for cost reduction while ensuring fairness and reliability in their decision-making processes.
The era of predictive modeling for cost reduction is upon us. It’s a powerful tool that, when wielded effectively, can revolutionize how businesses operate. Embrace predictive modeling as a strategic asset and pave the way for a more efficient, cost-effective, and competitive future.
Published By: Aize Perez